Published 10/4-2025 Læs dansk version
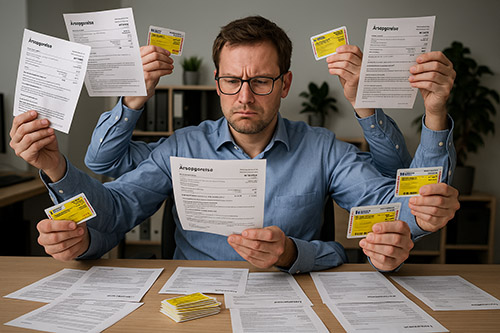
Dansk Folkehjælp wanted to optimize the application processes for the organization's aid programs, such as Christmas and holiday assistance for financially struggling families with children.
The organization was looking for a new system to classify applicants based on their tax statements and health cards.
To address this, Ergasio was integrated with Optical Character Recognition (OCR) models to extract unstructured data from tax documents and health cards.
This integration made it possible to automatically extract data from unstructured sources and transform raw data into structured formats suitable for AI-driven decision-making.
Automatic data extraction from unstructured documents and images
The system efficiently handles data originating from unstructured sources, such as applicants' tax returns and social security cards (sundhedskort), which are submitted as PDF and image files. By leveraging advanced Optical Character Recognition (OCR) technology, the system automatically extracts and transforms this raw data into structured formats, streamlining the application validation process.
Integration with external AI tool, OCR in Microsoft Cognitive Services
Ergasio's implementation includes integration with external AI tools like Microsoft Cognitive Services, enhancing its capability to efficiently process and validate applicant data. The Document Intelligence Studio AI model, trained in Azure Cognitive Services, scans various document formats, ensuring quick and accurate data extraction.
GDPR compliance ensured by installing Ergasio on the client's Azure cloud
To ensure GDPR compliance and safeguard sensitive applicant data, Ergasio is deployed on the client's own Azure cloud. This setup not only protects personal information but also maintains the stability and speed necessary to handle the high volume of applications during peak periods.
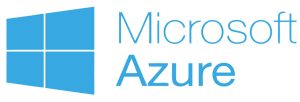
The Challenge
How to qualify an applicant?
The solution
Review the applicants’ tax statements and health cards. Unfortunately, this data exists in an unstructured format—either as PDFs or images.
Development
Switch2ai trained two machine learning models via Azure Cognitive Services.
Implementation
The solution was integrated into Dansk Folkehjælps existing application flow in conjunction with their web agency
Switch2ai has been incredibly efficient and super helpful in transforming our application process.
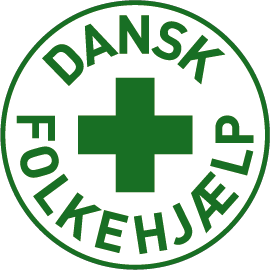
Lone Rasmussen
Chef for organisationssekretariatet
Implementation Details
With the implementation of Ergasio, applicants now upload their tax reports and children's health cards directly to the system. Validation occurs within seconds, significantly reducing the time and effort previously required. The Document Intelligence Studio AI model, trained in Azure Cognitive Services, efficiently scans tax returns (PDFs) and health cards (images, PDFs, HEIC, JPG).
Given the high volume of applications—nearly 30,000 during peak periods—the solution's stability and speed are crucial. Ergasio's deployment on the client's own Azure cloud ensures compliance with GDPR regulations, safeguarding sensitive applicant data.
Conclusion
The integration of Ergasio has revolutionized the way Dansk Folkehjælp's Aid processes applications, providing a seamless and secure solution that meets the demands of high-volume periods. By leveraging advanced AI technologies, the organization can now focus more on delivering aid to those in need, rather than being bogged down by administrative tasks.
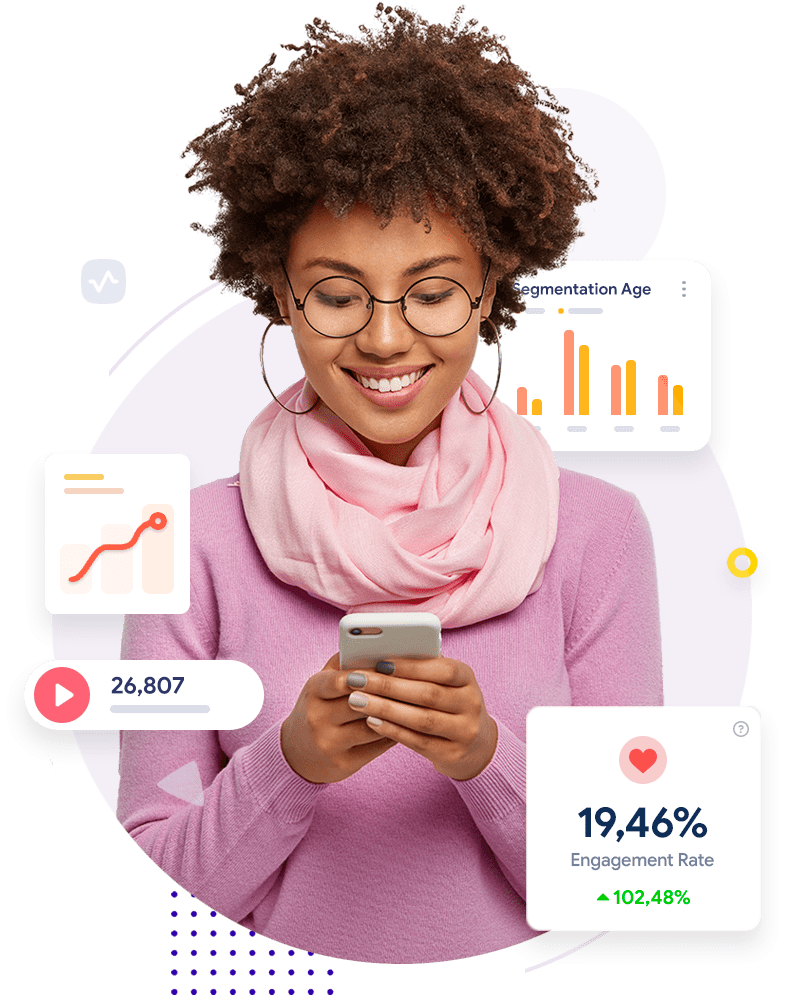
30k
Applicants log in to apply for grants - often within a short time frame when applications open
96k
Number of documents being uploaded, scanned and OCR processed by machine learning models
Want more information? 🙂
Get in touch with Ruvan Fernando for additional information. info@switch2ai.com or +4522860468